Oil and Gas Asset Data Quality and Asset Integrity
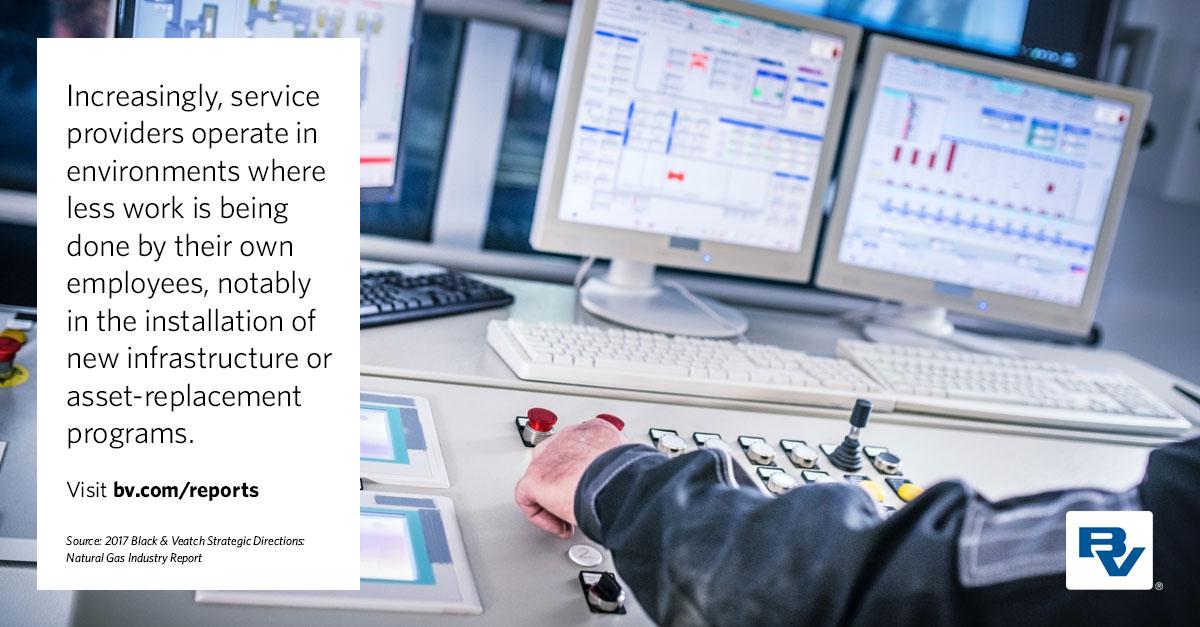
Oil and Gas Asset Data Quality and Asset Integrity
From the HAL 9000’s chilling psychosis to the world-ending artificial intelligence at the heart of the Terminator franchise, the movie industry has created a perception that data-driven computing is a heartless force singularly focused on results. Yet it is this singular focus that is critical to understanding the challenge facing the natural gas value chain when it comes to enterprise asset data quality. Today’s approaches to managing application data do not provide the high-quality foundation necessary to drive new insights into improving operations, regardless of how much we may wish for it.
Download the 2017 Natural Gas Industry Report
For more than a decade, risk-based approaches to asset management have been gaining prevalence across the utility space. Yet less than one-third of respondents use data to manage risk, with other key management functions like budgeting, strategic planning and daily operations even less reliant on data. This is despite current state and federal regulatory pressures pushing a drive to differentiate, quantify and improve asset data across the organization.
To understand the evolving role of asset data in the natural gas sector, it is useful to review the evolution of another mission critical data set. During the 1980s, major infrastructure sectors did not acknowledge the concept of “customers,” but viewed them as “accounts.” Actual usable customer data was limited at best-and often nonexistent. This perception was transformed over the last 20 years, with new applications like customer relationship management (CRM), Process Orchestration and a generation of customer intelligence solutions creating a precious resource, high-quality, integrated baseline data about customers and their preferences as predictors of future behavior.
The gas industry’s perception and management of critical asset data is in a similar transformative period, driven by high-profile failures of transmission and distribution assets, leaking storage facilities and, unfortunately, human tragedy. These events have driven new rules, process requirements and analytical needs requiring a greater understanding of a company’s enterprise assets that can only be achieved with new approaches, including data analytics built upon a solid foundation of asset data definition, capture and governance.
Three main types of asset data are necessary to improve operational performance. Structured and well-documented attribute data, coupled with conditional data, provide insights into an asset’s physical state. Unfortunately, conditional data such as leaks, breaks, corrosion, exposure, soil and road conditions, often is gathered only when an asset fails and needs replacing. Additionally, key inspection and observational data often is captured in an unstructured fashion (such as text comments) that, while generating a compliant record of work performed, fails to provide the structured, regimented capture approach needed to support new data analytics solutions.
These analyses are at the heart of future success in identifying trends and opportunities for asset replacement and overall gas network enhancement. While developing a comprehensive vision of system assets is broadly a goal of service providers, survey responses show that much work needs to be done. While nearly half the respondents claim good quality data, the statistics are undercut by the fact that availability and, more importantly, data completeness, are ranked much lower. Thus, organizations largely report they don’t have critical information they need to provide higher levels of service.
The lack of data completeness and accuracy can be tied to several factors, particularly access to assets and historical records. Unlike customer data, which can be updated by direct outreach, asset data can be hard to track. With much of gas transportation infrastructure underground or remote, often for decades, it can be difficult to collect and analyze efficiently. A significant group of respondents have not yet implemented solutions to improve data accuracy, completeness and availability.
The natural gas industry has done a reasonable job collecting historical asset data but lacks the type of data that can be used for predictive analysis. In many areas, data still is stored on paper or if in digital formats, any critical information is contained in comments fields or other notations where it cannot be automated and analyzed. If the raw data is mediocre, the information needed for analytics is in a poor state, limiting insights gained from even high-quality analytics software.
The sector faces an additional motivation for enhancing asset data quality. Increasingly, service providers operate in environments where less work is being done by their own employees, notably in the installation of new infrastructure or asset-replacement programs. Relying on third-party vendors increases the difficulty of gathering quality data. Business rules critical to the validation of source data on assets, typically not used by outside parties, need to be used to govern data-capture processes.
Addressing gaps in data quality will not happen overnight. The transformation of record-keeping has been underway for more than 40 years, and undoing the mazes created by mergers, acquisitions and asset software implementations will take time. Integrating functions of separate leak departments or technical services groups as well as the individual solutions and tactical programs will be necessary to create the nextgeneration enterprise asset data model. A complete picture of the history, condition and trending data for a company’s assets then can be developed, starting with recognizing that enterprise asset data is a business governance issue critical to future risk mitigation and business success.