Sustainable Networks: Saving Energy While Maintaining Customer Experience
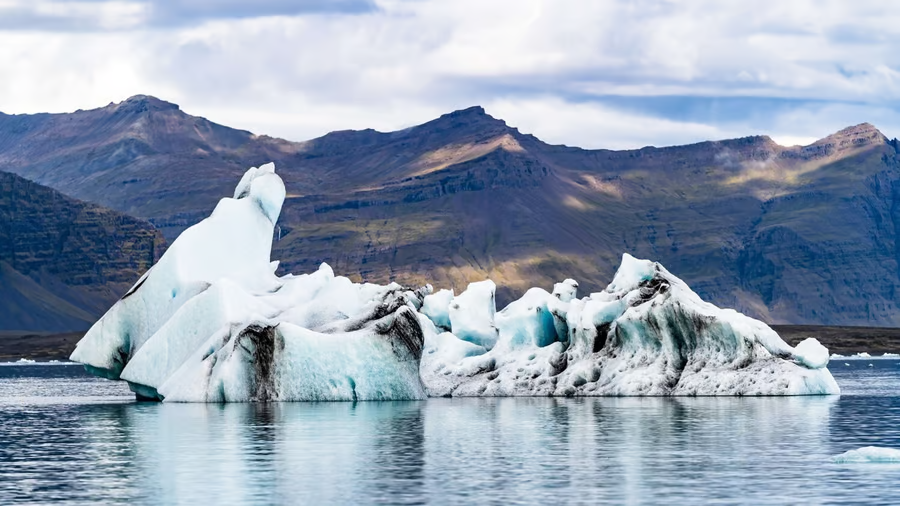
Annie Turner Journalist, Co-author
Gökce Alacadagli Service Portfolio Director, Energy Management Solutions
Reducing energy consumption while maintaining network performance is vital. That’s where optimized user experience and careful management of network performance comes into play. As part of our data-driven operations blog series, Gökce Alacadagli, Portfolio Director, Ericsson, talks to Annie Turner about innovative ways to cut power consumption in active and passive network elements.
In 2022, Ericsson published an industry white paper, On the road to breaking the energy curve . Its findings suggested that collectively operators were spending some $25 billion annually on energy. It became clear to Ericsson that the ever-rising curve of power consumption was not sustainable, economically or ecologically. Since then, Ericsson estimates that spending on energy could have risen above $30 billion due to soaring energy prices.
Gökce Alacadagli explains the curve in question is due to mobile networks being deployed to meet peak capacity demands, and with 2G, 3G, 4G and now 5G, the number of elements in the network is increasing. He says this means, “We need a more holistic approach. Sometimes, we focus too much on network KPIs and CapEx investments to expand the network and potentially increase overall energy consumption. This is even though most operators have communicated their group-level targets to become carbon neutral then net zero. This stimulates a mindset shift to transform from peak performance service provider to energy efficient – sustainable service provider.”
With all this in mind, the company developed a three-pronged plan to break the energy curve. The first is about evolving networks sustainably by “planning and investing in the right way, using spectrum most effectively; for example through powering Massive MIMO”, Alacadagli says.
The second concerns expanding and modernizing networks, such as replacing radio units with newer ones that consume up to 40 percent less energy. He explains, “This presents an opportunity to reduce energy consumption even though traffic is increasing due to more network capabilities.”
Alacadagli’s particular focus is on the third part: “How to operate today’s networks more intelligently, using AI and machine learning to reduce energy consumption now.” However, Ericsson has observed some hesitation to operating the radio access network (RAN) in a more power-efficient way in case the measures impact network KPIs which operators tend to assume will automatically damage customers’ experience.
Flawed perceptions
In fact, the reducing energy does not necessarily affect experience, or not perceptibly so – and Ericsson and others have transparent, empirical data to prove it. “If someone is watching a HD video on a mobile running at 28Mbps and the speed drops by 3Mbps, there is no impact on the performance of the video or the WhatsApp calls,” he states. “In fact, research shows most such consumer services can run well at an average speed of 10Mbps, which was also mentioned on our article Who cares about peak download speeds in 5G? earlier this year. So, if there’s controlled change in throughput due to energy optimization measures there will be no perceptible impact on customers’ experience.”
He continues, “We get very good data from the network and can help operators find the right balance between energy saving and optimizing throughput. Some parts of the network we don’t touch because customers don’t want to make any changes there, but in other parts a tweak of say 3 percent can result in a 10 percent saving.
“Previously our customers did not have the tools or solutions to safely explore different scenarios. Now we have a holistic solution for each part of the journey that we can show our customers and they choose their own scenarios.”
A blunt instrument
The simplest form of energy efficiency in any industry is putting energy-consuming devices in a low-energy or standby mode, “which we can do for radio nodes in multiple ways,” Alacadagli notes. “Multiple features do different things in the RAN and most vendors have software functionalities which turn the power amplifier on and off in microseconds.
“Low-energy-schedulers (LESS) schedule the data transfer to optimize energy savings, so that other hardware near algorithms such as Micro Sleep TX can become more efficient. MIMO sleep mode de-activates some of the branches and fast-sleep mode turns off the entire cell, especially on the capacity layer when traffic falls below a threshold. So, there is a foundation for energy saving in the RAN but due to operators’ anxieties about impacting network KPIs, they are generally only enabled at night. Here we propose solutions to manage them more intelligently, where we can implement different thresholds for day and night.”
Ericsson thinks there are huge improvements to be gained by exploiting accurate data to optimize energy usage without any noticeable changes to experience. Its ambition is to understand how energy can be used better as each network, cluster and region has different traffic volumes and varied user experiences.
“We must work with different protocols down to site or even cell level to maximize energy saving. Different sites have different traffic profiles, and we need to create an energy-saving pattern for them all,” Alacadagli says. “We propose cognitive solutions to watch traffic trends at sites or even individual cells and create policies and actions that can be applied individually. That’s the name of the game for energy efficiency across the entire infrastructure for telecoms networks.”
The power of passive infrastructure
He’s not just talking about active parts of the infrastructure, the radio units, either. As he points out, telecom sites need ‘passive infrastructure’ for the radio networks to operate 24/7. Passive infrastructure represents the support ecosystem to keep radio sites up and running, which constitutes batteries, power supply units and climate units to cool down the critical equipment. Alacadagli says, “Ericsson’s ambition is to empower cognitive energy management or holistic energy management solutions, using AI and machine learning, that can be applied to all technologies and all infrastructure, taking into consideration the traffic paradigms and user experience.”
In Ericsson’s view there are three main pillars that support this ambition: leveraging data to boost automation by pulling it from across the entire network; applying machine learning; then using the output to control of the network, end-to-end.
“We have element management systems and OSS pulling data that measures energy consumption and performance, so we know how each site is acting and the kind of customer experience they are providing, what kind of traffic they have and so on,” Alacadagli says.
“Also, we need to check and understand the data coming from the RAN to see consumption patterns and profile them. As a result of profiling – and not many operators do energy profiling for sites depending on the traffic they generate – we can benchmark sites for energy efficient from the gigabytes of data they provide to users.”
However, managing passive infrastructure generally involves truck roll to the site because, for instance, once the air-con unit is set to 21 degrees, it stays there. “If the passive infrastructure doesn’t generate data, we cannot control it; to control everything in this ecosystem, we need to invest in controllers, sensors and IoT devices to digitalize them. That's what we mean by maximizing the power of data to build automation.”
The gains are potentially huge, but so is the challenge of bringing passive infrastructure “into the fold” as originally no thought was given to including data from passive infrastructure in the mix, either by network equipment providers or passive infrastructure suppliers. Hence data from passive elements tends to be segregated. Customers need convincing of the return in the required investment, but Alacadagli is optimistic.
AI-based and data-driven
To achieve these ends, Ericsson’s launched its AI-based, data-driven approach to managed services, in 2020 as part of the Ericsson Operations Engine. The Energy Infrastructure Operations focuses on four areas Alacadagli explains.
Predictive Cell Energy Management application predicts traffic volumes and usage patterns, potentially down to each cell on a site to put them into optimal energy saving modes, like standby or sleep. Alacadagli enthuses, “We can use historic data to set parameters, or we can make real-time decisions and set new parameters, say every six hours. This is where things get interesting because generally, the parameters of these solutions are set, deployed and stay as-is, regardless of changing conditions. Alternatively, we can configure a node with different thresholds for different times of the day or put it to sleep mode immediately through closed loop automation.”
Energy profiling of sites and cells use algorithms to highlight, for instance, cells at some sites consuming more than comparable ones.
Anomaly detection and predictive infrastructure operations algorithms can be used to monitor the consumption of passive infrastructure – as well as radio elements – such as diesel generators, rectifiers and air conditioning units. If consumption is higher than expected, it can suggest a remedy. For example, it can ‘deduce’ that probably the air conditioning filter needs replacing, or it can remotely adjust the temperature settings for cooling optimization. This means the whole site – active and passive components – can consume less energy.
It is key that proactive management is in place at each site for the best results with power source optimization and to ensure, for instance, that there is enough battery capacity to come off the grid at peak times. Again, some operators are nervous of this routine shift to battery power (rather than seeing batteries as emergency back-up), but as AI and machine learning can review the best power source option every 15 minutes, attitudes are changing.
Transparency and proof
Gökce says, “If your solution and machine learning are transparent enough to show operators the kind of actions taken and the outcomes on a dashboard, it’s no longer about us selling them a solution. Being able to provide proof points is why have a lot of customer engagements right now.”
“The whole idea behind those four pillars is to use machine learning with different implementation methodologies, to take decisions at site or even cell level based on data from the sites. AI and machine learning become the primary tool to operate each side infrastructure independently at any given time and transform our industry regarding energy efficiency” Alacadagli concludes.