Bias in the Machine: How AI Fails Us and How We Can Fix It
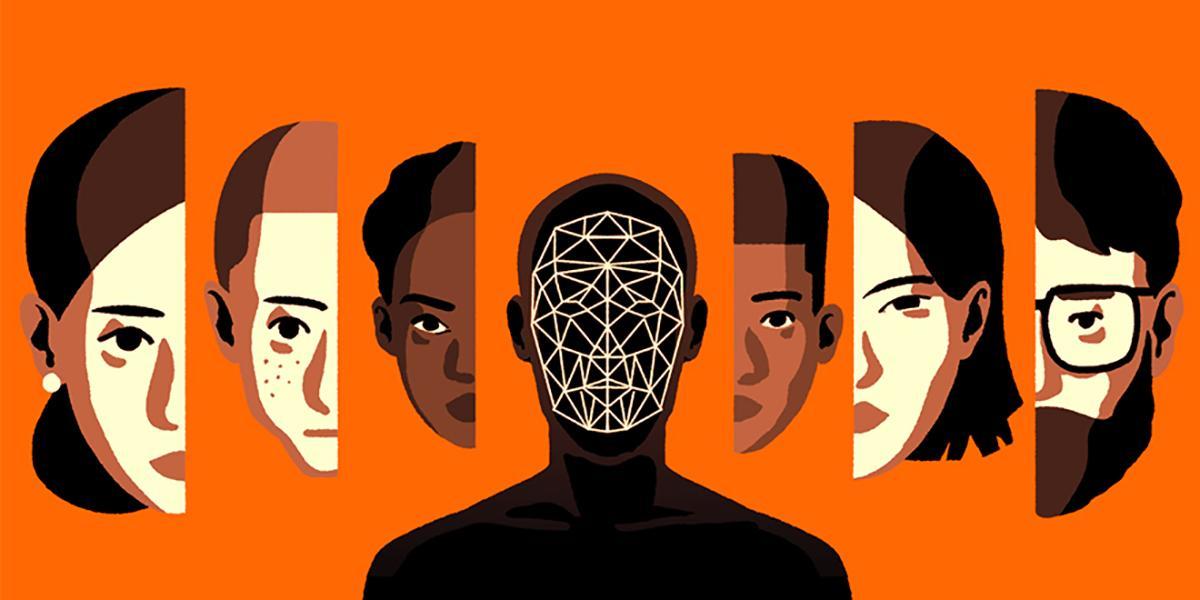
magine being jazzed to attend a performance by your favorite artist. But once you reach the venue's doors, you're turned away. The problem? It wasn't the security guards, but rather, the software, that made a mistake. That's because barcoded tickets have been replaced with face scans, and the algorithm in charge of admitting concertgoers doesn’t believe that you — are you.
This might be a serious annoyance, but companies like Ticketmaster are betting big on face recognition. And they aren’t the only ones interested in tapping AI to transform their businesses. As AI transforms the world, from autonomous vehicles to smart assistants to medicine-dispensing robots, the technology holds massive potential to do good, like solve crimes, speed up drug development and save the elephants. It also comes with unintended consequences, and the problem begins with data collected and used to train computers.
“Companies think AI is a neutral arbitrator because it’s a creation of science, but it’s not,” says Miriam Vogel, executive director of the nonprofit EqualAI, which works to identify and eliminate bias in AI. “It is a reflection of humans — warts, beauty, and all. This is a high-consequence problem.”
Most AI systems need to see millions of examples to learn to do a task. But using real-world data to train these algorithms means that historical and contemporary biases against marginalized groups like women, people of color and the LGBTQ community get baked into the programs.
“It’s humans that are biased and the data that we generated that is training the AI to be biased,” says Andrew Bolwell, HP’s global head of tech strategy and ventures. “It’s a human problem that humans need to take ownership of.”
Bias in many forms
The ways bias can reveal itself in different types of AI runs the gamut. Computer vision, the area of AI that deals with how machines see the world, is especially hazardous. Google Photos once tagged a photo of two black Americans as gorillas. In New Zealand, a passport system using AI didn’t believe that an Asian man’s eyes were open in a photo.
When it comes to written language, AI can misrepresent statements and entire groups of people. For example, the Turkish language uses “o” as a gender-neutral pronoun to refer to an individual, unlike English that relies on “she” or “he.” But because there are more examples throughout history of text about male doctors, Google Translate learned to take the Turkish phrases like “o bir doktor,” which literally means “the person is a doctor,” and translate it to “he is a doctor.” Google has since tried to reduce the bias in gender-neutral cases like this by offering up both masculine and feminine versions.
For spoken language, women and minority voices are less likely to be understood by speech recognition systems. Research shows that Google’s speech recognition is 13 percent more accurate for men than it is for women. Accents also lower accuracy rates, with Scottish accents tripping up a YouTube algorithm for automatically generated captions nearly half the time. Voice recognition technology used by the Australian government to test visa seekers for English proficiency found that an Irish woman with two university degrees didn’t speak her native tongue well enough to qualify.
How AI learns to discriminate
AI can also learn prejudices. In a machine learning product, Google included sentiment analysis capabilities that let computers decide whether a piece of text was negative or positive. A reporter found that when it was first released, the system considered statements like “I’m a gay black woman” and “I’m a homosexual” as negative sentiments. For a similar reason, YouTube’s algorithms have restricted content from LGBTQ creators or videos about LGBTQ topics, including videos for the It Gets Better campaign, which is designed to stop suicides among at-risk youth.
Human resource departments are also confronting bias in algorithms meant to automate hiring. Amazon shut down a recruitment algorithm that was found to penalize resumes that included the word “women’s” anywhere in the text. That might show up in accolades like “women’s chess club captain” or even naming an all-women’s college as an alma mater.
That’s because the AI was trained on resumes submitted to the company over a 10-year period, which mostly came from men, and the algorithm tried to replicate those results. Despite this example, more automated tools for hiring with additional features that will analyze mood or facial reactions are being created and put into use. Researchers like Aylin Caliskan, a professor at George Washington University who studies natural language processing, worry that bias that isn’t caught before it’s applied in recruitment practices like this could continue to harm underrepresented groups.
“It doesn’t just perpetuate bias, but it also becomes a feedback loop," Caliskan says. “It’s a very complex problem.”
Real-world risks
Bias in hiring and language censorship are serious issues, but the stakes get much higher when law enforcement is involved. Cities across the U.S. are looking to see how face recognition can empower law enforcement, modernizing efforts to track down criminals and close unsolved cases. However, civil liberty groups like the ACLU have raised concerns that AI technology isn’t advanced enough to use in law enforcement, and they have research to prove their point. In a test by the ACLU, face recognition software incorrectly identified 28 members of Congress, including civil rights leader and Rep. John Lewis from Georgia, labeling them as different individuals who had been arrested for a crime. Non-white members were found to be disproportionately misidentified.
These failings have meant some city governments are stepping in to stop the widespread use of AI in public services. In May, San Francisco became the first city in the country to ban the use of face recognition by municipal agencies, including the police, over concerns about privacy and racial bias.
Partnership on AI, a consortium of 80 tech companies, put out a report last month that said law enforcement should not use risk assessment tools when it comes to making decisions around jailing people, deciding bail, parole or probation. Already, half of American adults are in a law enforcement facial recognition database, and how it is used is completely unregulated at a federal level. Congress hasn’t passed digital privacy legislation since 1986, and has struggled to come up with a new law that fits the current technological landscape.
This opens the door for abuse and misuse as cities and states struggle to create a patchwork of laws. For example, in 2017, the New York Police Department used a photo of actor Woody Harrelson to search a database for a suspect — even though Harrelson had no connection to the situation — because officers thought the suspect and the celebrity looked similar.
Diversity is at the heart of the problem and the solution
White men make up the majority of the workforce creating AI, and the products they build don’t always account for — or work for — people who don’t look like them.
For example, when Joy Buolamwini was a computer science undergrad working on a facial recognition project, the technology she was working with couldn’t detect her dark-skinned face. In a 2017 TEDx Talk, she describes how she was forced to rely on her white roommate’s face to get it to work. Buolamwini brushed the issue aside until it happened again while she was visiting a startup in Hong Kong. During a demo of the company’s social robot, she was the only person in a group that the robot didn’t see.
For Buolamwini, these encounters with AI that couldn’t see her were due to the lack of black faces in the technology’s training sets. One of the most popular datasets for face recognition is estimated to be 83.5 percent white, according to one study. In her master’s thesis at MIT, Buolamwini found that three leading tech companies' commercially available face recognition tools performed poorly at guessing the gender of women with dark skin. In the worst cases, the odds were a coin toss, while white men had a zero percent error rate.
“These tools are too powerful, and the potential for grave shortcomings, including extreme demographic and phenotypic bias is clear,” she wrote in her testimony for the House Oversight and Reform Committee’s hearing on facial recognition technology last week.
In response to this threat, Buolamwini helped co-found the Algorithmic Justice League, a project based out of MIT that aims to highlight concerns around AI and design ways to fight back, through activism, inclusion and even art. She’s not the only one. Tech company employees are speaking out against working on systems they fear are ripe for misuse. Nonprofits like AI Now and EqualAI, are popping up to highlight the social implications of AI and create processes for better systems. Conferences like Fairness, Accountability, and Transparency are springing up to share work on how to make AI better for everyone.
The “pale male dataset,” as Buolamwini calls it, used by developers who are building these systems potentially exacerbates the problems of bias. A publication by AI Now gathered statistics on the lack of diversity in AI. Women make up 15 percent and 10 percent of AI researchers at Facebook and Google, respectively. At premiere AI conferences, only 18 percent of authors of accepted papers are women, and more than three-quarters of professors teaching AI are men.
The path forward is to diversify the inputs. The report argues that lack of diversity and bias issues “are deeply intertwined” and that adding more people to the mix will go a long way toward solving both problems. “If you put a team together with a similar background, you can end up with a situation where people agree without a lot of discussion,” says Alex Thayer, the chief experience architect and director for HP’s Immersive Experiences Lab. More diverse teams have a wider range of life experiences to apply as they think through and catch potential issues before a product is released.
Companies take action
Another part of the problem is the unwillingness of AI creators and users to question algorithmic output, according to Vogel. Developers of the technology need to be more willing to recognize its limitations.
Companies are starting to take that advice seriously. IBM, Accenture and Google have all released tools that can inspect algorithms for bias. Organizations like GLAAD are working with technology companies to offer their expertise and help in making products better and more fair. Done right, AI will overcome bias, according to HP's Bolwell. If systems are carefully designed and deployed in the right circumstances, AI could one day be part of making the world a more just and inclusive place.
“Until now, AI has perhaps been too human, revealing our shortcomings and faults,” Bolwell says. “But with an intentional approach, ‘artificial’ tools can be not only smarter and faster but also equitable and more inclusive.”