With AI Watching, Clues to Why Neighborhoods Flourish or Fail
AI imaging is delivering greater insights into neighborhoods than surveys and census data have ever been able to provide.
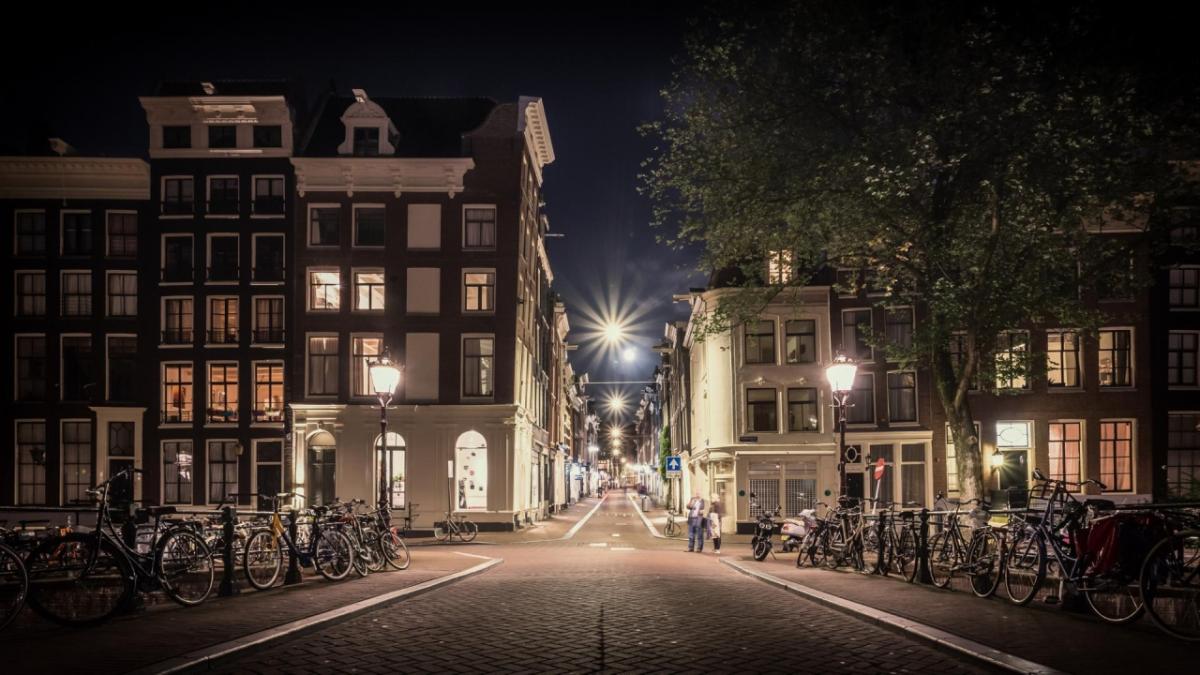
If residents of Brooklyn’s Prospect Heights neighborhood have a feeling their streets have gotten safer over the past decade, while residents of the Hillcrest area of Washington, D.C., feel less secure on several of their blocks, artificial intelligence has now delivered proof that their hunches are correct.
Using a new machine-learning algorithm they developed, researchers at Harvard and MIT combine photos from Google’s Street View service with human perceptions of a street’s safety to score whether a city block has improved or declined over time. The computer scientists and economists want their project, called Streetchange, to give urban planners artificial intelligence tools to help guide their thinking about evolving urban landscapes.
Given the demographic trends researchers foresee, policymakers will need all the help they can get: By 2050, over two-thirds of the world’s population is expected to live in urban areas. As that dramatic shift remakes society, it’s more critical than ever for urban planners to understand the impact and implications of that transformation.
Traditional data collection alone is not up to the task. Resident surveys are costly and take a lot of time to administer and process. Plus, the responses they elicit are notoriously unreliable. By comparison, Streetchange lets researchers study neighborhoods using high-quality, timely and granular data obtained from cameras atop roaming Google Street View vehicles, which is then rapidly and cheaply analyzed by AI.
A more useful view
Computer scientist Nikhil Naik, a Harvard University Prize Fellow and co-author of a study about the research, says Streetchange’s machine-learning algorithm has the potential to give U.S. policymakers and urban planners a much more useful window into the way demographic shifts impact communities.
Julia Lane, an economist at NYU’s Center for Urban Science & Progress, who wasn’t involved in the research, agrees. “This isn’t eye candy — it’s a very high-quality meal,” she says. “These people are thinking through what the measures they’re analyzing actually mean. It’s pioneering — a terrific piece of work.”
How the magic happens
The researchers initially trained their program in 2014 by feeding it images that had been tagged with the perceptions of more than 7,000 people who had been shown Street View photos of neighborhoods and asked which blocks looked safer.
Why tally people’s perceptions? A body of research has accrued over the years linking perceived neighborhood disorder with actual higher rates of criminality and poorer resident health outcomes. “[Turns out] the human decision of whether a place looks safe or dangerous is a good proxy for whether that street has improved or declined,” says Naik.
For the Streetchange project, the team had their algorithm chew on more than 1.5 million panoramic Street View images of city blocks in Baltimore; Boston; Detroit; New York City; and Washington, D.C., taken in 2007 and 2014. The algorithm compared the photos of the same blocks to measure whether they had improved or declined over those years.
The algorithm scored each image by placing its content into four categories: trees, streets, buildings and sky. Higher scores were assigned to photos with new buildings, repaved roads or more trees — elements that give people a relative feeling of safety. Then the score from the first year’s photo was compared with the score of the block’s image from the seventh year.
A score greater than zero meant a street’s perceived safety had improved from 2007 to 2014, while a negative number meant the street’s safety had declined. For instance, a row of new apartment buildings constructed in 2011 on Greenwood Street in the Dorchester neighborhood of Boston helped lift that area’s Streetchange score.
The factors that provide the biggest boost
The team didn’t stop at quantifying enhancement or decay. They also connected the scores of individual streets to administrative geographic areas called census blocks to seek out patterns using census data. They wanted to see which characteristics — population density, share of people with a college education, home prices, income, race and other factors — were most predictive of improvement for each block.
They found that three aspects help neighborhoods improve: education, location and the elusive “tipping point.”
First, they uncovered evidence that highly educated people living in dense clusters spurred neighborhoods to improve more quickly than other demographic factors, such as income, ethnicity and housing costs. This finding provides support for aspects of an economic theory called human capital agglomeration, in which people with higher education entice other people with higher education to move in — and infrastructure improvements follow.
The research also lent credence to a couple of controversial theories. First, the data indicated that neighborhoods with higher initial scores showed stronger signs of improvement from 2007 to 2014. This, the authors write, provides a tiny bit of support for the so-called tipping-point theory of urban change, which posits that bad neighborhoods get worse and good ones get better. While the Streetchange analysis did show that neighborhoods that started out better experienced more improvement over the study period, it did not find that neighborhoods that started out worse declined — they just improved less.
The researchers also saw evidence for an element of influential sociologist Ernest Burgess’ idea that distinctly different demographic and socioeconomic areas can “invade” other neighborhoods. Naik and his colleagues found that the neighborhoods they studied were more likely to improve when they were next to a city’s central business district or other neighborhoods that the algorithm had scored higher.
Better data = better decisions
Naik cautions that Streetchange isn’t meant to show cause and effect — a viewer can’t know, for instance, whether highly educated people moving to a neighborhood caused it to improve or whether improvements attracted more highly educated people.
Still, he notes that Streetchange could have an outsized impact in the developing world. In places where a census hasn’t been conducted for decades, for example, the tool could help aid agencies and government officials understand whether or not their work is having the intended impact on residents’ lives.
The AI approach could also shine a quantitative light on the growing problems of gentrification, economic inequality and related social issues. “How does ethnic and demographic composition change neighborhoods? How does immigration change neighborhoods?” Naik asks. “This is typically an area of research done with limited good data.”
Explore Streetchange’s maps of New York City; Boston; Detroit; Baltimore; and Washington, D.C.